What if your business could generate new ideas, content, and solutions—not just analyze existing data?
The answer is already here. According to McKinsey, 35% of global companies now use generative AI in their operations, with early adopters reporting up to 50% productivity gains in content creation and R&D. This isn't just automation—it's the dawn of AI-powered invention.
Generative AI represents a seismic shift in artificial intelligence. Unlike traditional AI that interprets information, these systems create—producing human-quality text, hyper-realistic images, functional code, and even breakthrough scientific hypotheses.
The market reflects this potential: Bloomberg Intelligence projects the generative AI sector to grow from 40 billion in 2022 to 40 billion in 2022 and 1.3 trillion by 2032.
Two forces are driving this transformation:
- Generative AI use cases and applications solving previously impossible problems:
- 61% of pharma companies now use AI for drug discovery (Accenture)
- AI-generated content accounts for 30% of marketing assets at Fortune 500 firms (Gartner)
- Generative AI development services that bridge the gap between potential and implementation:
- Custom solutions reduce deployment time by 60% compared to off-the-shelf tools (Deloitte)
In this article, we analyze five groundbreaking applications—from AI-engineered materials that cut manufacturing costs by 40% to financial models that predict market shifts with 90% accuracy.
You'll discover how industries are leveraging these tools not just to optimize but to reinvent their very foundations.
The question is no longer if generative AI will impact your sector but how soon you'll harness it.
Let's explore the real-world cases defining this revolution.
What is Generative AI and Why Does It Matter for Businesses Today?
Generative AI is a rapidly evolving branch of artificial intelligence designed to create original content such as text, images, audio, code, and video. Unlike traditional AI systems that analyze and classify data, generative AI models can produce new data based on their learned patterns. Popularized by tools like ChatGPT, DALL·E, and Midjourney, generative AI reshapes industries by driving innovation, boosting efficiency, and personalizing customer experiences.
So, how does generative AI work? At its core, generative AI relies on large-scale foundation models trained on vast datasets.
These models use deep learning techniques such as transformers and neural networks to understand context, style, and structure, allowing them to generate human-like responses or visuals. This makes them useful across countless use cases—from AI-generated marketing copy and product descriptions to synthetic images for design teams and predictive insights for R&D departments.
The applications of generative AI are transformative for businesses. Organizations can automate content creation, optimize workflows, and generate tailored communications at scale.
In industries like healthcare, finance, retail, and entertainment, generative AI is used to develop new drug compounds, create virtual assistants, design personalized shopping experiences, and more.
By adopting generative AI, companies improve operational productivity and gain a competitive edge in rapidly evolving markets. As the technology matures, expect it to become a core part of how businesses operate, innovate, and scale.
Whether you're exploring generative AI use cases or looking to integrate AI-generated content into your business, now is the time to embrace this game-changing technology.
Using Generative AI Tools to Improve Workplace Productivity in Marketing
How Does Generative AI Work? Understanding the Techniques Behind the Technology
Generative AI uses advanced neural network models—such as transformers, GANs (Generative Adversarial Networks), and VAEs (Variational Autoencoders)—to identify patterns in existing data and generate new, original content. Unlike traditional AI systems that process data or follow predefined rules, generative AI creates something new: images, text, music, videos, and code.
These capabilities are now powering real-world solutions across industries, from generative AI companies developing creative tools to enterprises automating content generation at scale.
Unsupervised or semi-supervised learning is a key generative AI technique that drives this technology forward. This approach allows models to learn from vast amounts of unlabeled or minimally labelled data, significantly reducing the time and cost associated with training. This enables organizations to build powerful foundation models quickly using publicly available or proprietary data.
For example, generative AI examples in business include AI-generated product designs, automated article writing, personalized marketing content, and even simulated customer interactions.
Many generative companies build tools that leverage these models to support product development, sales, and creative workflows.
Generative AI enhances conversational AI by improving natural language processing (NLP) and natural language generation (NLG).
Unlike traditional chatbots, which are limited to scripted responses, generative virtual assistants can understand user intent, respond contextually, and learn over time, delivering more human-like and meaningful interactions.
Ultimately, understanding how generative AI works helps businesses harness the full potential of this transformative technology.
Whether used in customer service, content creation, or R&D, these techniques are at the core of the next wave of innovation.
What are the Problems with AI in Business? Possible Solutions for Security
How ChatGPT, Gemini, Copilot, and Other GenAI Tools Are Revolutionizing Industries in 2025
Generative AI (GenAI) tools like ChatGPT, Google Gemini, Microsoft Copilot, and others are transforming how industries operate, making automation, content creation, and decision-making faster and more efficient.
From marketing and HR to finance, healthcare, and software development, companies are increasingly integrating GenAI to boost productivity, streamline operations, and improve customer experiences.
- ChatGPT by OpenAI has gained massive traction across sectors. With over 100 million weekly users as of early 2024, it's being adopted for everything from drafting emails to writing code and summarizing documents. In marketing, companies use ChatGPT to generate ad copy, blog posts, and social media content. In HR, it's assisting in resume screening and creating job descriptions. According to a McKinsey report, 79% of organizations have implemented, with ChatGPT being the top tool of choice.
- Google Gemini (formerly Bard) is Google's focus on productivity and content generation. Built to integrate with Google Workspace apps, it particularly appeals to organizations already reliant on Google Docs, Sheets, and Gmail. According to Google, Gemini was adopted by tens of thousands of enterprise customers within the first few months of its launch. It's especially popular in sectors like education and research, where document processing and summarization are frequently needed.
- Microsoft Copilot, integrated directly into tools like Word, Excel, and Teams, has become essential for enterprises using Microsoft 365. Copilot enhances productivity by drafting emails, analyzing spreadsheets, and generating PowerPoint decks. Microsoft reports that 40% of Fortune 500 companies have already begun piloting Copilot. In financial services and legal industries, where Microsoft products are standard, Copilot is accelerating workflows significantly.
Other GenAI tools include Claude by Anthropic, Jasper for marketing copy, Writer for enterprise content governance, and Synthesia for AI video production.
Industries use these tools to create scalable content strategies, automate customer support, personalize user interactions, and accelerate product development cycles.
According to Statista, the global market for generative AI is expected to reach $66.6 billion by 2025, with adoption surging fastest in the U.S., followed by the UK and India.
Nearly 52% of tech firms plan to increase GenAI investments next year.
As AI evolves, organizations benefit from productivity boosts and gain a competitive edge by leveraging data-driven insights and automated intelligence—making GenAI no longer a nice-to-have but a must-have.GenAI Tool | Primary Use Case | Industries | Features | Adoption Rate (2025 Est.) |
ChatGPT (OpenAI) | Conversational AI, content creation, code generation | Customer service, marketing, education, software | Natural language generation, plugins, code interpreter | Over 1.2M+ businesses |
Gemini (Google) | Search enhancement, code generation, data synthesis | Enterprise productivity, marketing, development | Deep search integration, multi-modal AI, factual updates | Estimated 800K+ enterprise users |
Copilot (Microsoft) | Coding assistant, integrated in GitHub/VS Code | Software development, engineering, DevOps | Real-time code suggestions, inline documentation | Integrated by 1M+ dev teams |
Claude (Anthropic) | Ethical AI conversations, summarization, Q&A | Legal, education, research | Constitutional AI for safe responses, privacy-first | Rapid adoption in research & law sectors |
Bard (Google) | Conversational AI, information retrieval | Healthcare, education, customer support | Search augmentation, question answering | Used by 500K+ professionals |
5 Surprising Ways Generative AI is Reshaping Industries
In this section, we'll get right in to five surprising generative AI use cases and applications that are redefining how businesses operate and how value is delivered.
Whether you're in media, healthcare, customer service, or creative industries, these examples will show just how far-reaching and transformative this technology has become.
Get ready to explore how generative AI is doing more than just generating text—it's reshaping the future of work and pushing the boundaries of what's possible.
#1. How AI is Powering Scalable Writing and Marketing With Automated Content Creation
Among the most publicized uses of generative AI is its ability to generate written content, computer code, and other kinds of text - a capability that falls under the umbrella of automated content creation.
The backbone enabling many automated content creation applications is the foundation model, which is a giant, multi-purpose machine learning model trained on a vast dataset. Foundation models can understand and generate language with human-level mastery through self-supervised learning. One prominent example is OpenAI's GPT-4.
In the media industry, generative AI is automating basic news stories and reporting through companies like Automated Insights and Forbes' AI tool Bertie.
The Associated Press uses AI to cover minor league baseball games. Generative AI can also write first drafts of content, which human authors then refine and edit to finalize.
This human-AI collaboration significantly increases individual productivity.
For businesses, generative AI can create all types of marketing and advertising copy tailored to specific campaigns, audiences, and media channels. Designhill used the Anthropic model to generate taglines and slogans for its customers. Generative AI can also produce product descriptions, website content, social media posts, email newsletters, and more.
Beyond text, generative AI shows promise for other kinds of creative content. Google Brain has developed Imagen, a text-to-image model that generates photorealistic images from natural language descriptions.
Anthropic has Claude, which produces logos from prompt phrases. Similar advances are happening with audio and video generation.
The upshot is that generative AI allows practically anyone to instantly produce volumes of customized, high-quality content. This disruption is forcing content creators and marketers to shift from purely manual methods to AI-assisted ones.
OpenAI's newest model, GPT-4o, may create coherent information with just a few demonstrative instances at rates ranging between 63.32 and 134.9 tokens per second.#2. How AI is Revolutionizing Pharmaceutical Innovation With Accelerated Drug Discovery
Drug discovery and development is an enormously complex and expensive process. It takes over 10 years and often more than $2.3 billion to discover, test, and launch a new drug.
A promising application of generative AI is radically accelerating the early stages of identifying and synthesizing new medicines.
One of the bottlenecks in drug discovery is exploring the astronomically large chemical space to find promising compounds. There are an estimated 1060 potential drug molecules—more than the number of atoms in the solar system.
Machine learning models can explore this vast space and predict molecular properties orders of magnitude faster than purely lab-based approaches.
Companies like Exscientia and Insilico Medicine use generative AI to discover new molecular structures and optimize their properties for specific drug targets. Their AI systems achieved in months what previously took years in the lab.
In 2021, Exscientia's AI-designed cancer drug entered Phase I human clinical trials in just 12 months - a record fast from design to trials. The drug was created through a technique called de novo drug design, where AI generates and tests theoretical compounds rather than just screening existing molecule libraries.
Similar AI techniques show promise for accelerating materials science and agricultural chemistry innovations.
Another benefit of AI is reducing the failure rate of drug candidates in costly late-stage trials. Only 13.8% of drugs entering Phase I trials eventually reach patients. By better predicting toxicity and efficacy early on, AI tools can focus testing on the most promising therapies.
Major pharmaceutical companies are now racing to tap AI, with AstraZeneca partnering with Exscientia and Sanofi collaborating with BlackThorn Therapeutics.
As AI continues maturing, the entire process of drug discovery stands to substantially accelerate.
#3. Realistic Synthetic Media: AI's Role in Creating Deepfakes and Digital Humans
There have also recently been incredible advances in a generative AI area called neural rendering that have never been able to synthesize realistic photos, videos, and audio. These apps are getting famous for their outputs, namely DALL-E 3, Stable Diffusion, and FakeYou.
Behind the scenes, these tools utilize generative adversarial networks (GANs). The two neural networks of a GAN compete against each other - one generates synthetic examples while the other differentiates real from fake.
This adversarial dynamic causes the outputs to become continually more realistic.
Initially, the generated images were blurry and cartoonish. Now, apps can render highly convincing faces and scenes from only text prompts at high resolution. For instance, prompt engineering techniques and inpainting leverage diffusion models like Latent Diffusion to edit faces or remove objects from photos.
Startups like ElevenLabs and Descript are creating models to copy anyone's voice from a few samples in audio. Synthetic voices are becoming very close to the quality of real human recordings. Audio forged could enable new applications for accessibility tools, entertainment, personal assistance, and others.
On the other hand, it also brings up the issue of voice spoofing and misinformation.
Within a few years, real video rendition may become a reality, known as synthetic video. Startups like Metaphysic.ai offer primitive face-swapping today.
However, the company trains its video models on extensive video datasets to produce fully artificial, photorealistic videos using text prompts or storyboards. Synthesia, the startup making its neural rendering platform available in the form of a media and gaming tool, has raised $50 million to grow.
Realistic synthetic video, known as neural rendering, may arrive within a few years. Startups like Metaphysic.ai offer primitive face-swapping today.
But models are trained on large video datasets to generate fully artificial photorealistic videos based on text prompts or storyboards. The startup Synthesia raised $50 million to grow its neural rendering platform for media and gaming.
As synthetic media quality crosses into "deepfake" territory, trust and ethics challenges will emerge in separating real from fake. Blockchain metadata and digital watermarking are potential technical countermeasures.
Nonetheless, generative AI marks a profound shift in democratized media production.
#4.Why AI Chatbots Are the Future of Support When IT Comes to Transforming Customer Service
Customer service stands among the most promising near-term applications of conversational AI.
Chatbots utilizing natural language processing, dialogue management, and generative capabilities are set to transform customer engagement.
Chatbots are available 24/7 to handle routine customer requests such as information lookup, account support, or placing orders. It relieves call center volume and allows human agents to solve more complex problems.
Above and beyond that, AI chatbots are forecasted to resolve up to 80 percent of customer service needs that can be achieved in only a few years.
Most importantly, today's chatbots are no longer limited to rigid scripts and are now becoming more like humans.
For instance, Constitutional AI, a startup that developed chatbots' self-supervision techniques, is an example of how chatbots are made helpful, harmless, and honest. The customer service chatbot of e-commerce platform Shopify is powered by its language model, Claude.
With further progress, generative AI can give chatbots imaginative abilities for open-ended dialogue. Apps like Character.ai and Sensorium's digital human platform aim to offer immersive conversational experiences. Lifelike avatars paired with empathetic listening and emotion-aware responses lead the way to relationship-based engagement.
Over the long term, virtually every business could have its intelligent voice or virtual assistant to engage customers. 5G networks, AR/VR interfaces, and voice technologies will expand access to AI chatbots from call centers to retail stores to smart speakers at home.
As chatbots grow more sophisticated, they create manifold opportunities to enhance customer satisfaction and brand loyalty while optimizing costs.
Companies failing to adopt AI for customer service risk a competitive disadvantage.
#5. The Rise of Creative Artificial Intelligence AI-Generated Artworks and Music, AI-Generated Artworks and Music
Machines excelling at human creative arts like painting, music, and storytelling occupy an almost mythical place in our cultural consciousness. Generative AI is turning this fiction into reality through models capable of making original art, sounds, and literature.
Apps like Midjourney, DALL-E 3, and Stable Diffusion indicate the early promise of AI to generate aesthetic imagery. By analyzing millions of photos and artworks, AI learns concepts about composition, lighting, color palettes, and more to produce completely novel images from text prompts.
The outputs mimic styles ranging from photography to impressionism to anime.
Developments in AI music composition are opening new pathways for creating original songs, instrumentals, and sound effects. For example, Anthropic has a Constitutional AI that composes music, while Google Magenta helps novice musicians write melodies. Endel creates personalized soundscapes to improve focus and sleep. As the technology matures, AI promises to augment human creativity across many musical genres.
In the literary space, apps like Anthropic's Claude, AI21's Jurassic-1 J, and Qualcomm's AI Model Inventor are crafting short stories and poems. Claude wrote a children's book using common-sense reasoning.
While the underlying quality remains uneven, AI is starting to exhibit glimmers of contextual awareness, humor, and emotional expressiveness.
Creativity was long considered a pinnacle of human intelligence. But as generative models ingest more training data spanning literature, news, scientific papers and human conversations, the outputs grow more sophisticated and multidimensional. Over time, AI could transform how stories are composed, from fiction to film to video games and beyond.
For commercial applications, brands working with influencers, photographers, and artists could utilize AI tools to ideate content strategies, compose unique music, or even co-create future advertisements.
Savvy companies will find opportunities to harness AI across their creative supply chain.
Wrapping up
So we explored how generative AI is revolutionizing industries—from automated content creation and accelerated drug discovery, to synthetic media, customer service transformation, and AI-generated art and music.
These powerful generative AI use cases and applications are just the beginning.
As businesses integrate generative AI into their workflows, they are achieving remarkable gains in productivity, creativity, personalization, and operational efficiency.
Yet, with innovation comes responsibility. Concerns around data security, bias, and ethical implications must be addressed proactively to ensure AI serves all of society fairly.
Many are asking, "Will AI replace everyone?" While some roles may be automated, the broader truth is that AI will more likely augment human capabilities, creating new opportunities and reshaping roles rather than eliminating them.
According to a 2024 Gartner report, 70% of businesses using AI tools like ChatGPT, Gemini, and Microsoft Copilot have reported enhanced decision-making and efficiency, with nearly 60% planning to expand AI investment in the coming year.
So, how will AI change the world in the next 10 years?
We can expect breakthroughs in healthcare, education, logistics, and customer experience—empowering businesses and individuals alike. Generative AI is not just another tech trend; it's a transformative force akin to electricity or the internet.
Organizations that embrace this technology strategically, ethically, and boldly will lead the way in a world reshaped by intelligent innovation.
We think you might like to read next
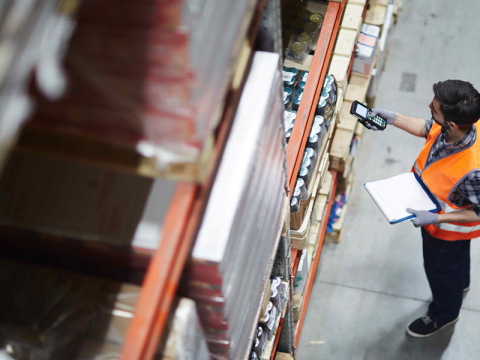